NVIDIA Fast-Tracks Custom Generative AI Model Development for Enterprises NVIDIA Blog
The challenge lies in ensuring that growth is profitable and that users generate profits once they sign up and stick around for a long time. Generative AI is already demonstrating its potential to revolutionize industries, with models like Stable Diffusion and ChatGPT setting historical records for user growth. The market https://www.metadialog.com/healthcare/ is witnessing an influx of startups developing foundation models, AI-native apps, and infrastructure. Generative AI models typically require large training data to produce high-quality outputs. Generative AI models, such as GANs (Generative Adversarial Networks), can create new content, including images, videos, and music.
Moreover, validating data integrity and coherence is vital before feeding the preprocessed data into the LLM. Verify the consistency in labeling and ensure that the data accurately reflects the intended task or domain. Address any remaining inconsistencies or errors to safeguard against potential biases or misinformation that may impact the model’s training.
Author & Researcher services
It learns from historical data trends, correlations, and insights throughout training. After training, it incorporates into business operations, automating processes, forecasting results, and making data-driven suggestions. By ensuring accuracy and relevance through ongoing monitoring and feedback, numerous enterprise areas can benefit from wiser decisions, improved processes, and innovation. In this layer, relevant algorithms are chosen, neural network designs are designed, hyperparameters are tuned, and models are trained using labeled data.
Or you need additional logic as part of your prediction like pre or post processing for example. Earlier in this article, we briefly touched upon the cost of in-house versus outsourcing AI management. Hiring an in-house team is the more expensive option when considering salaries, recruitment, training, and benefit costs.
Why would a customer services business choose to use a Custom LLM over an Open Source LLM?
You can create a personalized ChatGPT chatbot for your business by feeding Botsonic your data by following the steps below. Finally, install the Gradio library to create a simple user interface for interacting with the trained AI chatbot. You see, by integrating a smart, ChatGPT-trained AI assistant into your website, you’re essentially leveling up the entire customer experience. Identify the goals and outcomes you plan to achieve, along with listing the challenges.
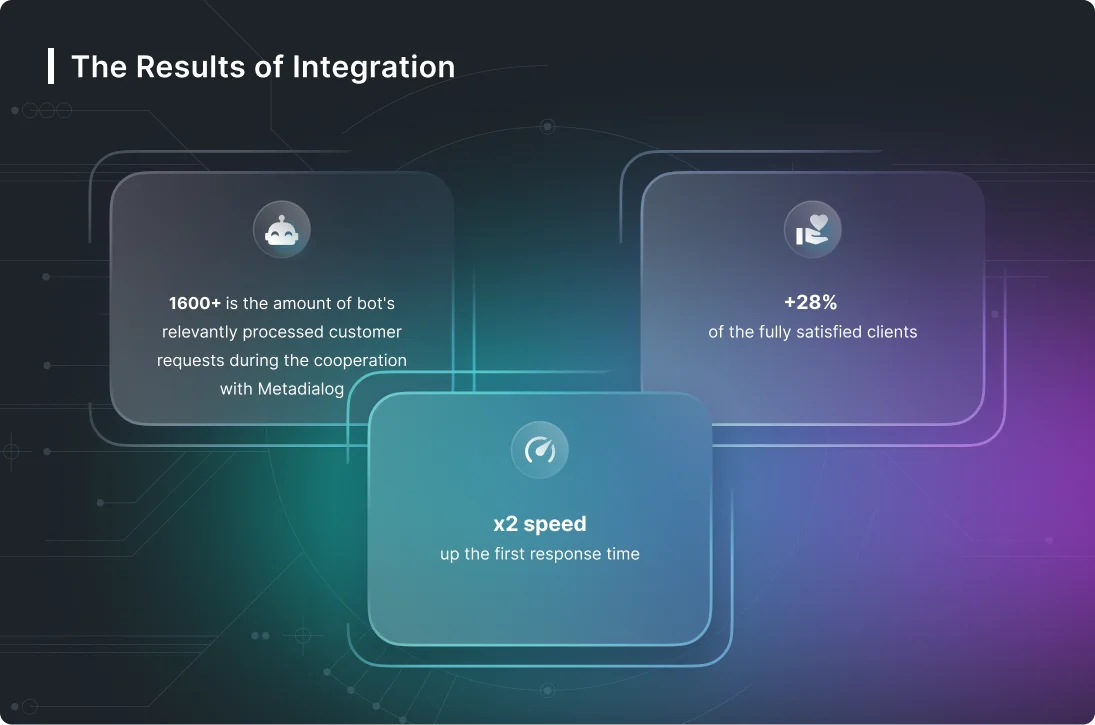
Overfitting can be managed through proper regularization and hyperparameter tuning. They provide a more personalized and efficient customer experience by offering instant responses to user queries and automating common tasks. Custom chatbots can handle a large volume of inquiries simultaneously, reducing the need for human teams and increasing operational efficiency.
Safeguard data privacy and security
Furthermore, residents benefit from improved access to healthcare services, personalized health monitoring, and timely alerts regarding disease outbreaks. Healthcare Information Systems contribute significantly to disease monitoring and management, enhancing public health outcomes within the smart city infrastructure. Using techniques and technology, epidemiology is mostly used to determine the degree of cognition from biomedical data. Big data applications in health coverage are a rapidly expanding topic, including numerous recent results and approaches reported in recent years. In particular, increased operations in genomics let researchers conduct novel genetic sequence correlation analyses of disorders. Commercial intelligence helps healthcare professionals use the enormous amounts of client records gathered to make wise judgments.
Before embarking on custom training for your LLM, clearly defining its purpose and scope is crucial. Whether it’s a question-answering system for a knowledge domain or another application, this definition will guide the entire development process. Once the task or domain is defined, analyze the data https://www.metadialog.com/healthcare/ requirements for training your custom LLM. Assess the availability of domain-specific data; is it readily accessible, or will you need to collect and preprocess it yourself? With the task and data analyzed, set clear objectives and performance metrics to measure the success of your custom LLM.
Cleaning and Preprocessing the Data
Before starting on a custom AI journey, it’s worth researching the market to see if there happens to be an application out there that already does precisely what you need. Although it would be rare for that to be the case, there’s little point in re-inventing the wheel. Explore ecosystem of ready-to-use Supervisely Apps that work on top of deployed neural networks and supercharge various aspects of labeling with AI.
No comment yet, add your voice below!